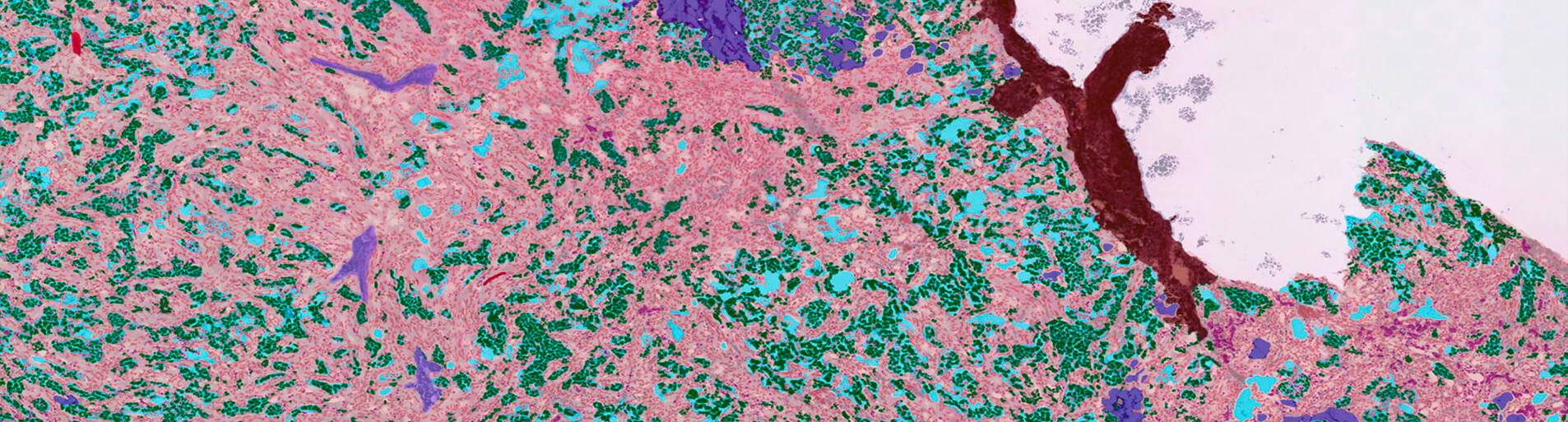
Data Science & AI
The Data Science & AI research unit focuses on optimizing data collection processes in clinical care settings, constructing expansive research databases like the IJB Breast Cancer research database, and implementing multicentric data collection frameworks. These efforts enable the aggregation of big data and facilitate federated learning techniques, preserving patient privacy. Additionally, the unit develops AI-driven applications, including auto-segmentation tools and quantitative image analysis pipelines, and focuses on quality assurance initiatives for reproducible radiomics image analysis. As a third pillar, we are dedicated to the development of prognostic tools that aid oncology experts in making informed clinical decisions. To achieve this, we focus on multi-modal data, including anatomical and molecular imaging, digital pathology, clinical data and genomics, bringing together the expertise from various departments and research laboratories within our hospital in large multidisciplinary research projects. The global ambition of the Data Science & AI research unit is to improve patient care and outcomes, through the implementation of a learning healthcare system and the application of artificial intelligence and big data analysis.
A selection of our projects:
Selected Publications:
Real-time motion management in MRI-guided radiotherapy: Current status and AI-enabled prospects.
E Lombardo, J Dhont, D Page, et al. Radiother Oncol, 109970. (2023) DOI:10.1016/j.radonc.2023.109970
Pre-trial quality assurance of diffusion-weighted MRI for radiomic analysis and the role of harmonisation
Z Paquier, SL Chao, G Bregni, et al. Phys Med, 130;138-146. (2022) DOI:10.1016/j.ejmp.2022.10.009
Radiomics software comparison using digital phantom and patient data: IBSI-compliance does not guarantee concordance of feature values
Z Paquier, SL Chao, A Acquisto, et al. Biomed Phys Eng Express, 8(6). (2022) DOI: 10.1088/2057-1976/ac8e6f
Machine learning model using F-18-FDG PET/CT-based biomarkers to support clinical decision making in advanced chemorefractory metastatic colorectal cancer.
J Dhont, H Levillain, T Guiot, et al. Eur J Nucl Med Mol Imaging, 49(1);S242-243. (2022) DOI: 10.1007/s00259-022-05924-4
A selection of our projects:
- Imaging Score in chemorefractory metastatic colorectal cancer
- AI for radiotherapy target optimisation in early-stage breast cancer
- ARTEMIS: Artificial Intelligence for tailoring in early mammary cancer – individual systemic therapy
- Optimisation of Lu177-PSMA treatment in metastatic colorectal cancer
Selected Publications:
Real-time motion management in MRI-guided radiotherapy: Current status and AI-enabled prospects.
E Lombardo, J Dhont, D Page, et al. Radiother Oncol, 109970. (2023) DOI:10.1016/j.radonc.2023.109970
Pre-trial quality assurance of diffusion-weighted MRI for radiomic analysis and the role of harmonisation
Z Paquier, SL Chao, G Bregni, et al. Phys Med, 130;138-146. (2022) DOI:10.1016/j.ejmp.2022.10.009
Radiomics software comparison using digital phantom and patient data: IBSI-compliance does not guarantee concordance of feature values
Z Paquier, SL Chao, A Acquisto, et al. Biomed Phys Eng Express, 8(6). (2022) DOI: 10.1088/2057-1976/ac8e6f
Machine learning model using F-18-FDG PET/CT-based biomarkers to support clinical decision making in advanced chemorefractory metastatic colorectal cancer.
J Dhont, H Levillain, T Guiot, et al. Eur J Nucl Med Mol Imaging, 49(1);S242-243. (2022) DOI: 10.1007/s00259-022-05924-4